PERMANENTLY REMOVING A GOOGLE BUSINESS LISTING FOR A CLOSED STORE: STEP-BY-STEP GUIDE
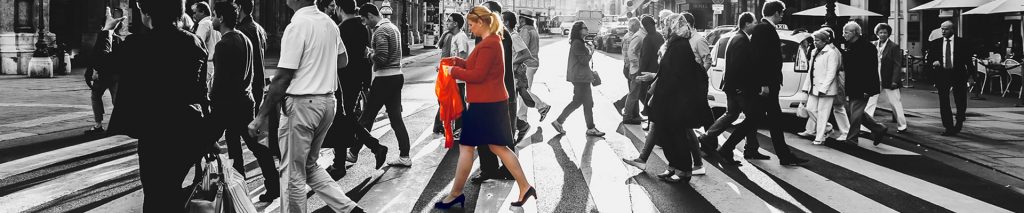
If you’re managing a Google Business Profile (GBP) for a store that’s permanently closed, it’s essential to ensure that the listing is accurately removed from both Google Search and Maps. Unfortunately, the process isn’t as simple as clicking a “delete” button. To guide you through this often misunderstood process, here’s an authoritative and complete step-by-step […]
HOW TO IMPROVE GOOGLE REVIEWS FOR RESTAURANTS: A COMPREHENSIVE GUIDE
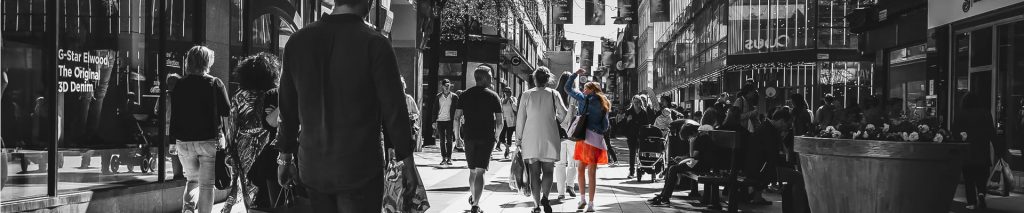
Google Reviews are a vital element in shaping the online reputation of your restaurant. With potential Guests relying heavily on these reviews to make snap (“zero moment”) dining decisions, improving your Google Reviews can directly influence your restaurant’s sales growth & overall success. Here’s a step-by-step guide to effectively enhance your Google Reviews, backed by […]
HOW TO CREATE A HIGH-PERFORMANCE FUNNEL IN META ADS BY CONSUMER JOURNEY STAGE <br />[STEP-BY-STEP GUIDE]
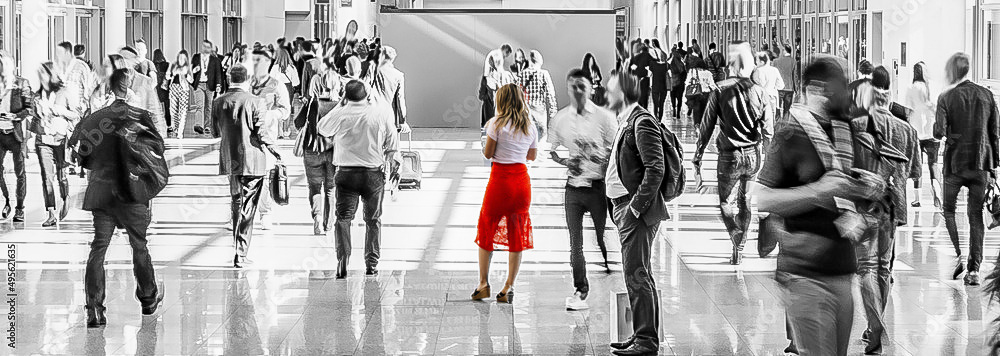
INTRODUCTION Creating a high-performance funnel in Meta Ads that targets various stages of the ConsumerJourney requires a structured, intelligent approach and an equally well thought implementation in Meta Ads Manager. Here’s a detailed Step-by-Step Guide to help you set up higher performing Multi-Touch Funnels using Meta Ads. WHEN TO USE A MULTI-TOUCH FUNNEL If you’re […]
MASTERING THE NEW 2024 EMAIL DELIVERABILITY STANDARDS: STAKING YOUR PLACE IN GMAIL AND YAHOO SUBSCRIBER’S INBOXES
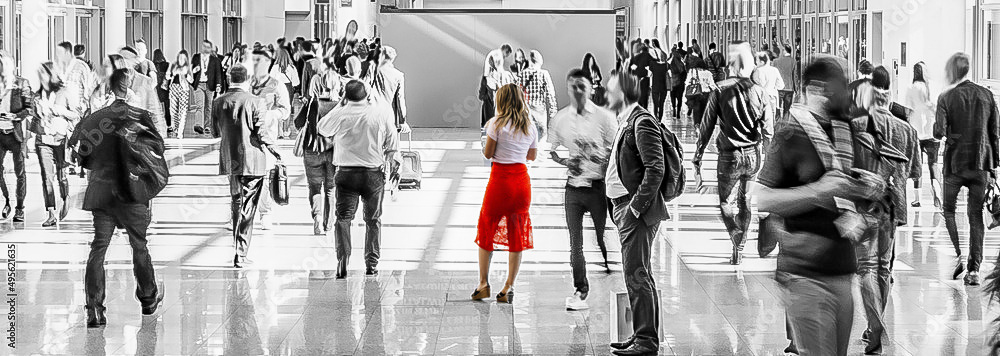
In the ever-evolving landscape of email deliverability, staying ahead of the game is non-negotiable. Google and Yahoo’s stringent new rules are hardly recommendations —they are a mandate. The Good News: BuyerGenomics has been tracking these changes since their inception ―and has you covered ― all the way to the inbox. Keep reading to learn more […]
The 3 Immutable Laws of Email Marketing
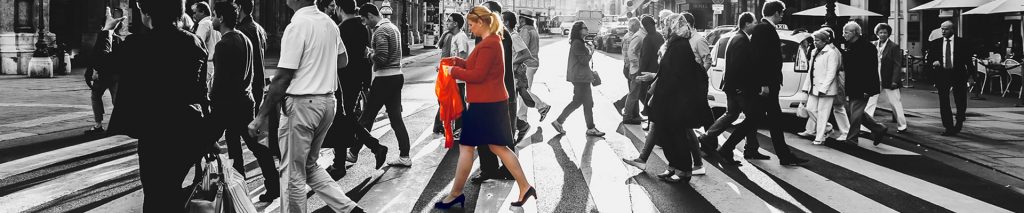
Email marketing remains a cornerstone for businesses seeking to connect with their audience. However, mastering this art requires an understanding of the fundamental principles that govern successful email campaigns. In this blog post, we’ll delve into the three laws of email marketing that not only make your emails relevant but also ensure they are welcomed […]
What Meta Advertising is Best for Driving Sales Growth?
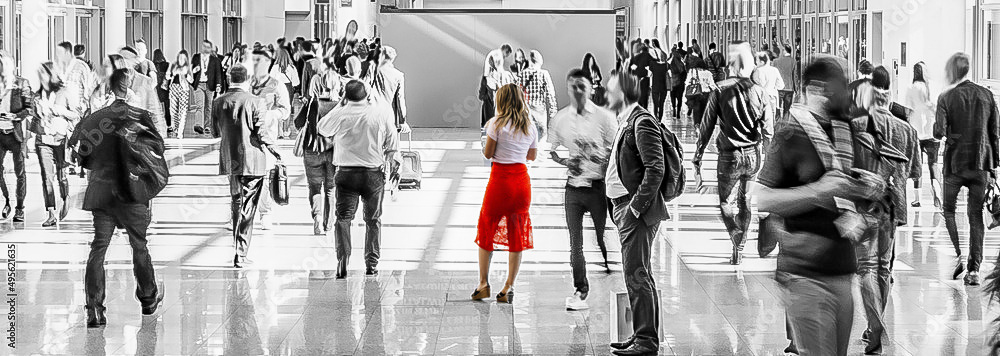
Rather than a complex and overly nuanced answer to the question of which Meta advertising is best for driving sales growth, let’s get right to the point. For brands seeking the maximum impact on sales growth, the best advertising option is generally Meta Ads (formerly known as Facebook Ads.) Meta Ads Are Considered Best for […]
Unlocking Customer Sentiment: BuyerGenomics’ HeartBeat Revolutionizes Insights
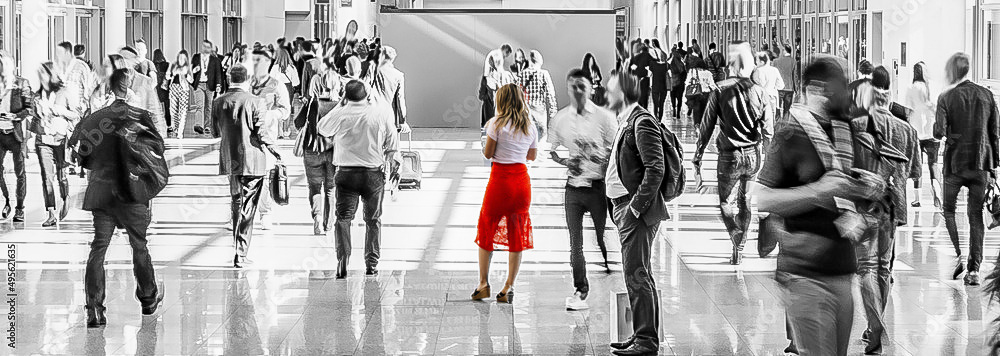
Welcome to the BuyerGenomics Thought Leadership Blog, where we’re excited to introduce our groundbreaking HeartBeat feature, designed to revolutionize how you understand and engage with your customers. In this post, we’ll delve into the HeartBeat tool’s capabilities, benefits, and how it can elevate your customer intelligence game. Introducing HeartBeat: Your Fast Track to Making Customer […]
HOW GOOGLE MEASURES STORE VISITS…<br />(AND IS IT ACCURATE?)
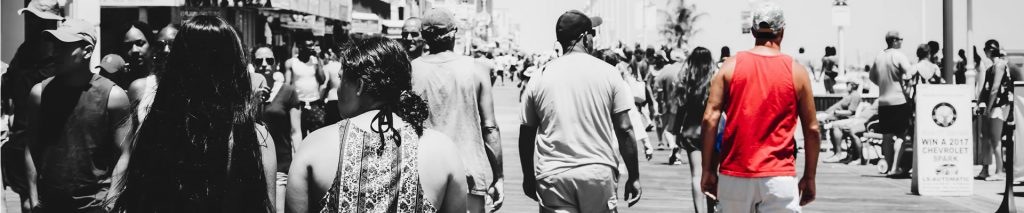
A huge number of brands rely on Google search ads to drive in-store traffic. However, some brands question the accuracy of the Google Ads attribution. Google uses AOV (“average order value”) ―a plug variable that the marketer provides. Some consider this measure inferior to pure online tracking of an online sale, which measures actual AOV […]
What percentage of sales should my marketing spend be?
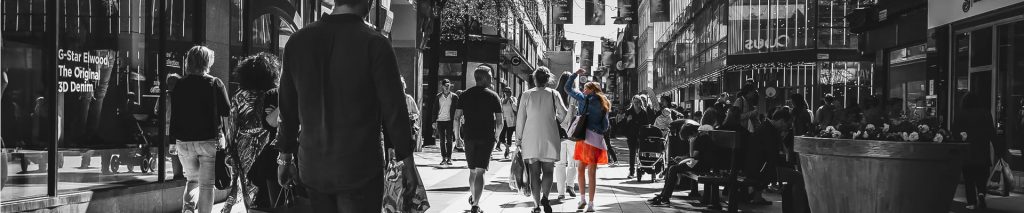
An eCommerce Manager recently posed this question… “My CFO is saying my spend of 10% is too high, This excludes development, hosting and salaries. Including salaries we’re spending 15%. So what percentage of revenue is your ad spend ―including ad agency fees, any consumer shows and photography?” TLDR: It depends on your goals and the […]
The Full Price Customer: How To Get & Keep Them
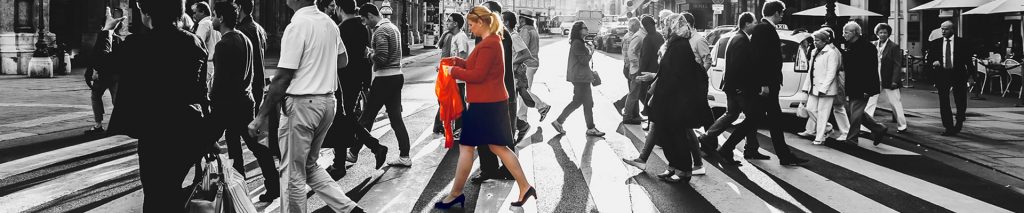
The refrain from retail CMO’s has been consistent and almost deafening. They say: “We don’t just need more customers, but the right customers.” “We need to grow margins.” “We need to reduce our dependency on discounts.” Even during this year of economic recovery, luxury brands in particular have been seeking to improve margins and sales by selling more full […]