Personalization is about the Person
At its core, 1:1 marking is about people – not products or channels. In fact, not just “people,” but individuals.
But the truth is, most marketers treat consumers as one-dimensional commodities, or worse – extensions of their last product purchased. This leads directly to improper personalization with irrelevant messages, which both squanders potential leads and drives away existing customers by training them to ignore brand messages.
Therefore, it is critical to clarify exactly what constitutes properly executed, holistic personalization, and precisely how brands can provide the best customer experience through such efforts.
When done right, personalization becomes a core component of marketing success. Simply put, relevant messages sell better.
Proper Personalization
These days, consumers have come to expect – and even demand – a wholly comprehensive, personalized experience. Econsultancy recently referenced a consumer survey that found 64 percent of respondents now expect an individualized experience, while 85 percent consider an individualized experience to be important.
With so many brands battling for their attention everyday across a wide array of channels, customers feel that they deserve to be viewed and addressed as individuals with a pulse – not just faceless components of larger masses.
Meanwhile, marketers are constantly sifting through vast data heaps in an attempt to get to know their customers better and generate truly personal relationships.
However, even with greater technology, mathematical abilities, and more available data than ever before, they are still making key mistakes along the way. Such mistakes wind up taking a heavy toll on Customer Acquisition Cost (CAC) and Return on Investment (ROI).
Prevent “Pesky” Personalization
Few daily life annoyances rank higher than receiving unwarranted or irrelevant messages or pitches from anyone – let alone marketers.
Nowadays, if you are going to ask a consumer to spend his/her valuable time and money purchasing your product, make sure you are speaking to the right person, at the right time, with the right pitch, and via the right channel.
If not, the best case scenario is that those messages are simply ignored. At worst, a slew of impertinent messages could turn customers off from your brand altogether.
Refine Your Personalization Engine
In order for personalization engines to run properly, all ingested data must undergo an extensive refinement process. This requires a degree of commitment and awareness that many companies lack.
Truthfully, there is a great deal about customers that marketers simply do not know. Even what we call Big Data consists of many holes and gaps, while most personalization engines are only built to act upon available data (or “known information”).
In turn, when marketers stick to targeting the customer only with data already available to them, they wind up blitzing some poor individuals with messages until they simply cannot take anymore. This is particularly unfortunate due to the drastic disparity between the amount of people with “known” behaviors and the rest who are unaccounted for.
In fact, the former generally make up less than 5 percent of the total accessible universe. Therefore, under such circumstances, 5 percent (known) are unnecessarily harassed, while the remaining 95 percent (unknown) are completely neglected.
Surely, we can do better than that.
Transform “Unknowns” to “Opportunities”
Enter segmentation and modeling techniques. Various forms of segmentation is a common technique deployed by many marketers to categorize and prioritize various cohorts and sub-groups of their respective customer base.
One common statistical method of segmentation is cluster analysis, which creates distinct segments by mathematically optimizing similarities and differences among the target audiences. Typical results of cluster analysis are any number of distinctive groups that share common demographic and behavioral characteristics.
Once the grouping is complete, each of these segments can be further described with the members\’ aggregated demographic profiles, life stages, interests, and – most importantly – their collective behavioral profile and outlook regarding specific products or services.
While segment-based profiles may not yield explicit descriptions of each individual, they provide important insights about the target base, and guide marketers for better personalization in the beginning stages of such endeavors.
Beyond Segmentation
Segmentation is a long-established, familiar practice that continues to play a vital role in marketing. After all, it certainly is much more effective than using raw, unorganized, and unfiltered data for customized messaging. Or even worse – doing nothing at all.
With segment descriptions at their disposal, copywriters can message as if they are speaking to the target personally. Undoubtedly, designing copy for a segment full of people described as “Comfortable Golden Years” or “Up and Coming Young Suburban Households” would be much easier than doing so for unknown, faceless targets.
However, this traditional process does have its shortcomings. For instance, many segments can be far too broad – lumping loads of people into cohorts who may share some demographic commonalities, but not necessarily all of the same behavioral characteristics.
These types of issues become much more visible when such a “descriptive” tool is employed for targeting purposes. An above-average index value of a particular characteristic within a segment does not rationalize treating thousands (or even millions) of people in a target group the same way.
For example, not everyone inside a “luxury” segment is necessarily driven towards luxury cars/vacations, or high-end fashion/jewelry. Even among luxury buyers, their affinity towards specific types of products would not be simply distinguished by basic segmentation.
After all, every individual has multiple predominant characteristics. We are not so simply homogeneous, which is why – if the goal is to create a truly holistic customer experience – it becomes necessary to dig into more detailed profiles on an individual level.
Producing Personas
To understand individual targets more comprehensively in situations like these, gaps in data must be filled through statistical modeling techniques.
Remember, even in the world of Big Data, it is still impossible to know everything about everyone – and many organizations lack the ability to both collect data properly and harness useful insights from collected data.
One of the most important roles of modeling in this data-rich environment is to summarize complex arrays of information into “answers to questions.”
Examples of such questions include:
- Who is more likely to be a cutting-edge technology buyer?
- Who is more likely to buy children’s products?
- Who is more likely to be into fashion?
- Who would be a full price purchaser?
- Who would be a bargain seeker?
- Who is more likely to be a repeat customer?
- Who is more likely to respond to particular type of offers (e.g, free shipping vs. 15% off)?
Therefore, in order to obtain the most comprehensive, 360-degree customer view possible – even with incomplete sets of data – certain types of statistical modeling methods must be implemented. Personas – in comparison to simple segments – would allow marketers align better with their target customers.
Propensity Scores
A customer-centric data view is achieved when all transactional, demographic, geographic, and behavioral data is centered around “each” customer or prospect. From there, such “event”-level data would be transformed into “descriptors” of individuals, which would then lead to model scores that would provide answers to specific questions.
Comprehensive individual-level descriptors detail – beyond demographic profiles – personal spending patterns including categorical purchases, browsing history, amount/consistency of purchases, price levels across each channel/category, and sets of time series variables neatly aligned around each person.
Then, through modeling techniques, such information will be further converted and summarized into forms of “personas” – or propensity scores – commonly expressed (for simplicity) on a scale of 1 to 10.
For example, if the question is to see each individual’s propensity to be an “Early Adopter of Technology,” all users would have to know is that a score of 10 would indicates “highly likely to be an early adopter,” without having to go through a series of seemingly unrelated data points laboriously.
This is where your analytics engine kicks into a higher gear and works to fill in the holes of unknown territories in your customer base. In this way, “everyone” in the target universe is viewed in a much more detailed, nuanced manner.
For instance, while all people are inclined to be “discount-buyers,” but not necessarily to the same degree. Only a certain amount of people are willing to wait in line all night for a television sale on Black Friday. Clearly, those people would score highly in the “bargain seeking” category. In a case like this, if a decision maker has access to a persona called “Bargain Seekers,” such intelligence would be readily available to treat target customers differently and more effectively.
And there is plenty of room for other personas, as well. With multiple persona characteristics for each person, you are able to identify each of their predominant traits (or highest/lowest scores). In fact, depending upon the marketers’ needs, it is entirely possible to have any number of personas for an individual.
Naturally, some people may score highly in multiple categories simultaneously. With standardized score format (1-10, for example), marketers – or even machines – can easily rank persona scores within one target, at the time of offer. If a target scores high in both Early Adopter and Frequent Traveler categories, which one should win out? This actually represents extra opportunities where the marketer would link up multiple sustainable offers for each target, and rotate them over time.
Ultimately, marketers will be able to increase coverage of their personalization efforts by employing personas that are available for most targets – not just depending on “known” information with much scarcer coverage.
With such a sophisticated scoring system, machines can be trained to send the best messages to the right customers at the right time, granted that a content library is also aligned with such personas – as in what personas should see what creative version. Consider the possibilities if done for millions of people in real-time.
Segments vs. Personas
To answer a common question regarding the key differences between segments and personas, below is a chart contrasting clustering/segmentation methods against model-based personas.
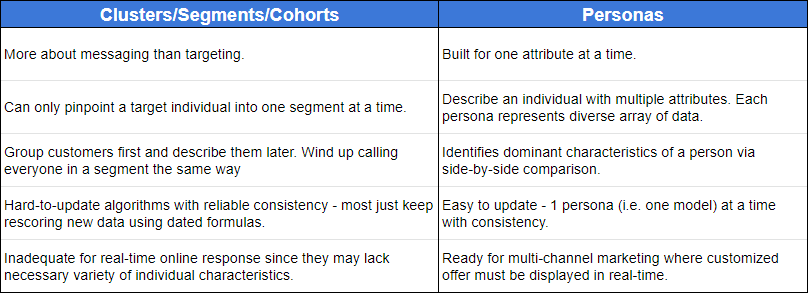
Since they are designed for one particular behavior or proclivity at a time, personas are quite versatile and flexible. On the other hand, segments are more rigid by design, as they are grouped into limited numbers (at times predesignated numbers) of segments – typically anywhere between 10-70. There, each individual is forced into one segment at a time.
These technical differences lead to huge differences in usage. For instance, targeting would be less precise with segments, since each segment might be too large or too small, mixed in with multiple conflicting traits among their members (e.g., not everyone in the “Luxury” segment will respond to a specific product offer the same way). With personas – or with any model scores – users can finetune the size of the target using specific models depending on the purpose of marketing campaigns (e.g., target with “Luxury Vacationer” model, mixed with “Likely to Response to Last Minute Offer” and “Fall Cruise Season” models).
For messaging, the notion that each segment or persona should be linked to a particular version of creatives is the same. Since lack of creative version is often the biggest bottleneck in an organization, using segmentation may seem suitable in most cases.
However, for more diverse dynamic contents (especially for personalization of inbound traffic) personas would be much more suitable due to their built-in flexibility. Equipped with an array of personas for “each” visitor, once the identity of the visitor is revealed, the content can change based on one or more dominant characteristic of the target. Even a machine can be tuned to identify such dominant traits of a person in real time, because personas by design can be compared side-by-side – for one target at a time.
Flexible design also makes any necessary updates or adjustments much more seamless. For instance, if certain model scores appear to be drifting away from your original design, simply update the anomalous ones – not the entire stack of personas.
Conversely, it is quite challenging to update segments using clustering techniques while maintaining similar segment characteristics that users are accustomed to. However, to be effective, models and segments must be updated with newly available information periodically.
With the continuous evolution of AI and machine intelligence capabilities each year, the whole process could actually be automated – from updating models to sending omnichannel messages.
Conclusion
Ultimately, the end goal of personalization is to decisively gauge which types of messages and product offers best appeal to your multifaceted customer base.
Although marketers are generally more familiar and comfortable with it, the practice of segmentation is insufficient on its own. For one, segments are fundamentally designed to create broad, generalized message groups – not individual-level personalization scores with deeper sets of variables.
Meanwhile, model-based personas offer a nimbler and more adjustable approach that helps deepen customer relationships through relevant messages designed to fit the persona profile, leading to higher chances of conversion.
Practically, marketers must rethink the way that they assess customer data altogether. Doing so requires dedication and coordination as a company, with a strict adherence to the chief objective of fully actualized, individualized personalization.
While some parts of this endeavor may utilize machine intelligence, it should be guided by humans to ensure proper individual-level data management, target setting and analytical work for model development, and proper deployment of persona-based omni-channel messaging – leading to an optimal customer experience (actual processes to be illustrated further in future articles).
Essentially, a fully personalized, relevant customer experience facilitates trust, increases loyalty/retention, makes customers feel important, and further drives sales. This is the most critical role of advanced analytics in a time of plentiful, but never complete data.